In this decision, the EPO Board of Appeal provides a comprehensive technical and legal overview of a neural network “as such”.
Object of the Invention:
- the application relates to a neural network (NN) apparatus
- claim 1 differs from the closest prior art in that the different layers of a NN are connected in accordance with an error code check matrix
Examination Division:
- distinguishing features do not serve a technical purpose and do not relate to a specific technical implementation
- distinguishing features pertain to initial, fixed structural definition of an abstract mathematical neural network-like model with unknown input and output data
Appellant (part I):
- claimed NN apparatus has a new and non-obvious structure
- claimed NN solved a technical problem by providing effects within the computer related to the implementation of NN (storage requirements)
Board (part I):
- the network structure only defines a class of mathematical functions which, as such, is excluded matter
- while the storage and computational requirements are reduced in comparison with the fully-connected NN, this does not translate to a technical effect, for the simple reason that the modified NN is different and will not learn in the same way
- the NN requires less storage, but it does not do the same thing
- for instance, a one neuron NN requires the least storage, but it will not be able to learn any complex data relationship
Appellant (part II):
- NN generally solve technical problems by automating human tasks
- NN apparatuses are artificial brains and that artificial brains solve an automation problem, because they can carry out various complex tasks, instead of the human, without being programmed specifically for one task or another
Board (part II):
- no evidence that NN functions like a human brain
- whilst the functioning of NN may not be foreseeable prior to training and the programmer may not understand the significance of its individual parameters, the NN still operates according to the programming of its structure and learning scheme
- the claims do not further specify any particular task, i.e. type of relationship to be learned, for the NN à NN does not solve any specific automation problem
Appellant (part III):
- a technical problem may also be solved if the outputs of the system have an implied further technical use (G 1/19, reasons 137)
Board (part III):
- claimed learning and use of the NN “to solve a classification problem or a regression problem” can use any data
- outputs of the NN do not have any implied “further technical use”
- outputs may be related to forecasting stock market evolution
Conclusion:
This decision provides a technical background regarding NN (reasons 7 to 8.2) and a legal background regarding NN (reasons 9 to 11.3), which provides a good insight into this topic.
Furthermore, the below figure shows according to G 1/19, point 85 and 86 how and when “technical effects” or “technical interactions” based on inter alia non-technical features may occur in the context of a computer-implemented process (the arrows in the figure above represent interactions and not abstract data). In this decision T 702/20 it was discussed whether the NN/ non-technical features contribute to the technical character of the invention via the output side, via the input side and via the technical implementation.
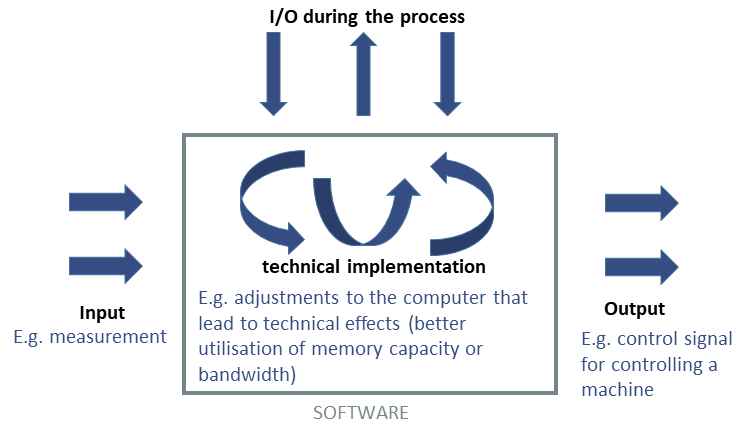