This decision is about the output of a machine learning algorithm. The output of the algorithm is more accurate here compared to the prior art. However, this is not a reason that the output automatically serves a technical effect. The output therefore does not automatically lead to non-technical features making a technical contribution via the output.
Object of the Invention:
- the present application is concerned with the generation of billing codes to be used in medical billing, wherein billings are provided to an insurer for reimbursement
- computer-based support systems have been developed to guide human coders through the process of generating billing codes
- claim 1 specifies a computer-implemented method for improving the accuracy of automatically generated billing codes
Board I (inventive step):
- a billing code is non-technical administrative data
- generating a billing code is a cognitive task
Appellant (inventive step):
- use of machine learning techniques to improve the accuracy of the machine output
- invention is technical because it improved the system so that it would generate more accurate billing codes in the future
Board II (inventive step):
- if neither the output of a learning-machine computer program nor the machine output’s accuracy contributes to a technical effect, an improvement of the machine achieved automatically through supervised learning for producing a more accurate output is not in itself a technical effect
- in this case, the learning machine’s output is a billing code, which is non-technical administrative data
- the accuracy of the billing code refers to “administrative accuracy” regarding, for example, whether the billing code is consistent with information represented by a spoken audio stream or a draft transcript
- the learning machine to generate more accurate billing codes or, equivalently, improving the accuracy of the billing codes generated by the system, is as such not a technical effect.
Conclusion
Furthermore, the below figure shows according to G 1/19, point 85 and 86 how and when “technical effects” or “technical interactions” based on inter alia non-technical features may occur in the context of a computer-implemented process (the arrows in the figure above represent interactions and not abstract data). In this decision T 755/18 it was discussed whether the non-technical features contribute to the technical character of the invention via the output side and also via the technical implementation (although the latter is not discussed here in this commentary).
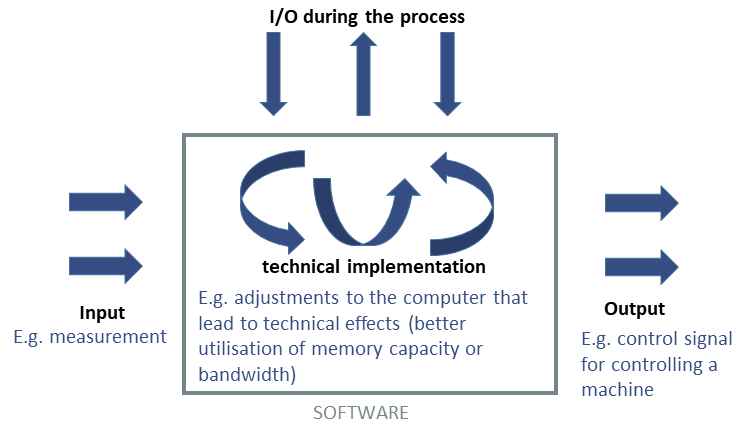